Here at Natural Intelligence Systems we have built a new Neuromorphic machine learning system that addresses many of the problems experienced with today’s machine learning algorithms.
We’ve summarized these problems below. If you’re experiencing any of these, you should take a look at the Natural Intelligence system!
1. You’re spending too much time data wrangling.
Are you spending the bulk of your time cleaning and preparing data for use in machine learning pipelines? Do you spend too little time on the fun stuff of selecting and optimizing your machine learning models?
2. You’re having trouble gathering enough training data.
Do you find you have difficulties optimizing your machine learning models because you do not have enough data? Is getting adequate data too expensive, time consuming, or even impossible to acquire?
3. Your current models don’t handle drifting data.
Do you need to take models out of production because accuracy is dropping due to changing conditions? Do you have to constantly retrain your models?
4. You need to explain why.
Are you being asked to explain the model’s decisions because of new regulatory constraints and cannot? Do you trust your models results?
5. Your model misses new classes and anomalies.
Are you struggling with results that are clearly anomalous but aren’t predicted as such?
6. You struggle with noisy data.
Does accuracy fade when system sensors get noisy or start to fail? Is it essential that your system is insensitive to perturbations or spoofing attacks?
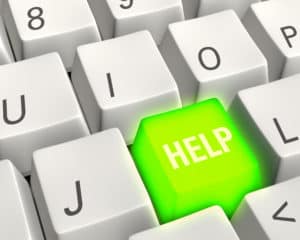
If you are struggling with these challenges you should take a look at the next generation Neuromorphic Machine Learning (NML) system that is available as a Platform-as-a-Service (PaaS) offering from Natural Intelligence Systems.
The Problem with Today’s AI
Today’s deep learning systems are built upon well understood multi-layer deep neural network (DNN) models. These foundations have enabled the strong growth of the technology but are now sources of some fundamental weaknesses of DNN models. These models use complex mathematical methods to find optimal weights. This requires huge training data sets and computational horsepower. They can also be brittle when it comes to identifying new items.
Learning new patterns continuously while performing inference is not practical using today’s neural networks. These neural networks are unintelligible to humans, and therefore both the training and test results remain unexplainable.
The dense representation of features used in DNN models causes these systems to be vulnerable to noise. Even a single change to an input bit may totally change the network’s behavior and cause false positives.
The “curse of dimensionality” dictates that any increase in feature-dimensions must be accompanied with an exponential increase in the number of training samples to maintain equivalent sampling distance.
Natural Intelligence: A New Frontier in AI
In contrast, Natural Intelligence’s NML system uses a pattern-based model that does not use complex math. It is modeled after the brain’s neocortex – enabling it to learn quickly with small amounts of data. Like the neocortex, it receives a stream of data and different “neurons” react when patterns are recognized, with every neuron determining its next action in parallel with every other neuron.
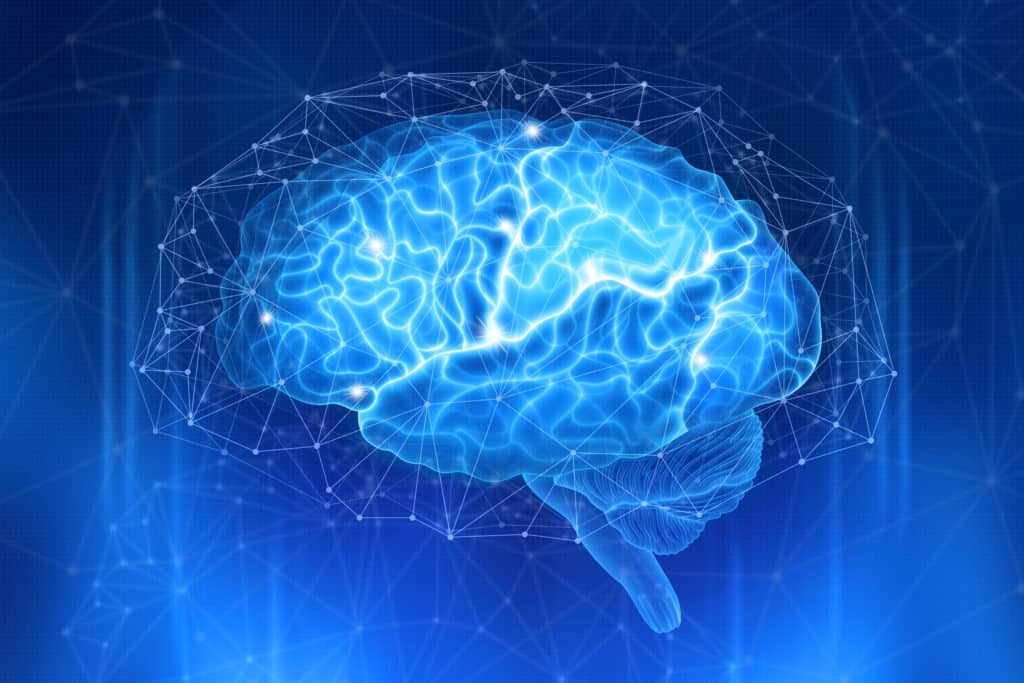
Continuously learns
Because the NML model is fast and forgoes the back propagation of existing models, it can learn continuously, track drifting data or even learn “new” or anomalous classes in addition to the classes it initially trained on. As a result, supervised, unsupervised, or dynamic learning (unsupervised clustering within a trained model) are all made possible.
Explainable AI
The NIS model is thin (not deep like neural networks) and maintains the semantic information of the pattern from input to output. Model predictions to be “explained” so that every output signature can identify the input conditions that produced the result. The NIS model’s explainability is vitally important for verification, validation, and for building trusted autonomy in the system.
Resilient to Noisy Data
The NML model handles messy data because it uses sparse vectors for data representation. These vectors are mathematically resilient to perturbations at the input. In addition, NML uses a sparsely connected model of learning based on Hebbian principles to strengthen or weaken learned synaptic connections on every data observation, quickly converging to recognize patterns using very little data and with few epochs.
With all of these advantages why continue to struggle with your old machine learning model. Consider evaluating the capabilities that the Natural Intelligence Neuromorphic Machine Learning system can bring to bear on your machine learning challenges. Contact us today to learn more.